Google’s Deepmind has taken another step forward in creating a computer that is able to outthink humans, or at least match them. In a recent paper, researchers revealed that the deep Q-network (DQN) was able to match expert human players in a majority of Atari 2600 games.
Previously computers have demonstrated an ability to beat humans at chess and Jeapordy. DQN however is not a supercomputer but an algorithm which can be run on any computer. Additionally, by focusing on multiple games for a console rather than a single game, researchers are better able to track the progress of the algorithm over time.
According to the new paper, published in the journal Nature, DQN was able to play 22 better than an expert game tester. It did worse than humans at 20 and essentially tied at the remaining 7. In an earlier paper, published in 2013, DQN took on human opponents in just seven games, winning at only three of them.
While the accomplishment is impressive, DQN still has quite a bit of work to do before truly matching up with humans. The AI algorithm has a memory that lasts only four frames of video, or one-fifteenth of a second. As a result it has a limited ability to strategize or plan. The games that it does well at tend to be those where the reward for an action was almost instantaneous.
As a result of its limitation, DQN excelled at games like “Space Invaders” and “Breakout” but didn’t fare well at games like Ms. Pac-Man. The game was unable to plan ahead when navigating the mass and failed to learn that “magic pellets” allowed you to defeat the ghosts and simply avoided them as part of its strategy.
DQN’s version of long term memory involved storing “memories” of actions and reactions from the game fed back into its decision making process. This is a bit different from what we think of as memory because it is not so much a collection of past events as a statistical analysis of probabilities based on past events.
According to the team behind the AI, expanding the attention span and memory of the software is one of the current priorities. The team is also working to make it capable of a more systematic approach to games, rather than its current random hit or miss approach.
DeepMind is currently working on having DQN play games for early PCs and Super Nintendo to introduce it to simple 3D environments. The exploration of those environments is intended as the bridge to real world environments.
“Ultimately the idea is that if this algorithm can drive a car in a racing game, with a few tweaks it will be able to drive a real car,” said Demis Hassabis, head of DeepMind at a press conference Tuesday according to MIT Technology Review.
The goal of DQN however is not to improve Google’s self driving cars, at least at this stage. Instead it is aimed at Googles best known, core, products such as search, translation and mobile assistants.
“Imagine if you could ask the Google app for something as complex as, ‘Okay, Google, plan me a great backpacking trip through Europe,” said Cassabas.
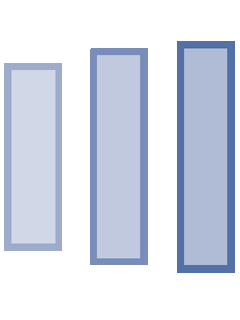
is a